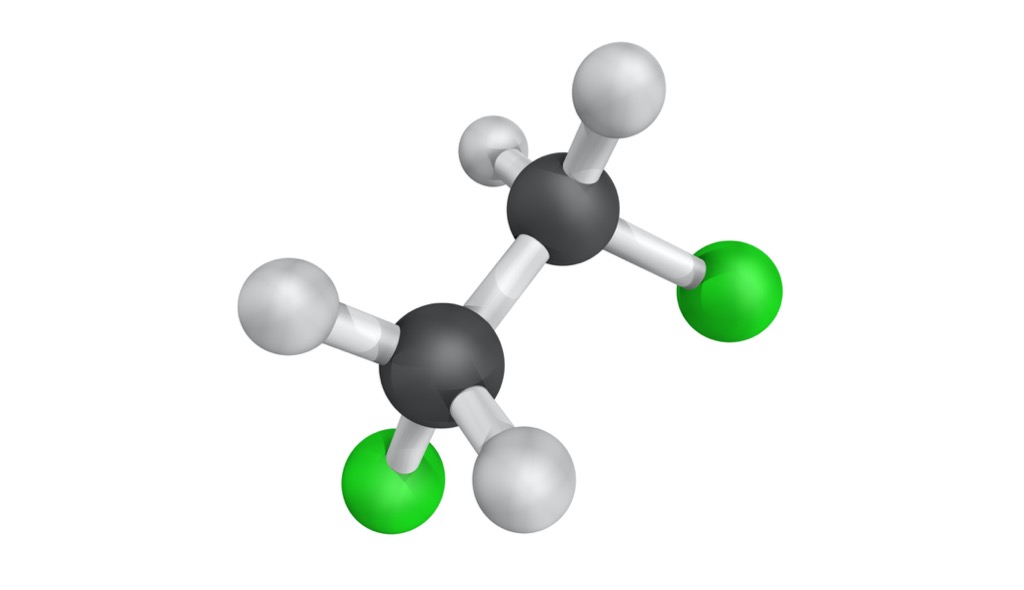
Quality and yield losses are a perennial problem for most chemical manufacturers. Some just see this waste as the price of doing business. Others, like the global manufacturer in this case study, used customized AI to prevent these losses from happening in the first place – saving one million euros annually in the process.
For chemical manufacturers, production losses can come in many forms: quality variabilities, impurities, incomplete reactions, losses during separation and purification, etcetera, etcetera. These production losses are costly, hurting manufacturers’ bottom line and sucking up precious time and resources from their manufacturing teams.
But what if you could prevent these losses from occurring in the first place? That’s what one global manufacturer of Ethylene Dichloride managed to do — saving nearly one million euros each year on a production line that, until then, had been suffering significant annual losses.
The primary problem was a toxic side product (trichloroethane) that kept forming during the production process. Despite the best efforts of the company’s process experts, the cause of this process inefficiency remained frustratingly elusive for years.
Generic AI and Analytics Tools Make No Sense
Part of the problem was that the production process in question was relatively complex, with some 4,400 data tags. Advanced as they were, the analytics tools used by their process experts still limited them to conducting ad hoc analyses of a select number of tags. This was of little help, since once their existing theories were exhausted, they had no idea where to continue looking for the root cause of the problem!
Due to the significant financial impact of this inefficiency, the company decided to invest in Industrial Artificial Intelligence. But attempts to use generic AI solutions still failed to uncover useful or accurate results, due to the complexity of their production process. These solutions were simply not made for continuous manufacturing, and were therefore unable to cope with the unique complexities of the process and resulting data.
As their VP Manufacturing noted, “These vendors touted some powerful AI technology. But continuous chemical manufacturing processes like ours produce uniquely complex and messy data that their algorithms simply didn’t understand. So, the end results either made no sense or were clearly inaccurate.”
Finding The Root Causes For Actionable Insights That Make Sense
To solve complex continuous manufacturing process inefficiencies, one must first pinpoint the specific problem.
To begin, an automated root-cause analysis can reveal the hidden causes of a manufacturer’s losses. Based on these results, the manufacturer can be provided with predictive recommendations to reach their optimal process settings to avoid losses and maximize capacity. Finally, proactive alerts will enable their manufacturing teams to prevent losses before they occur.
In the end, the company’s process experts were finally able to pinpoint the primary causes of their yield and quality issues, and were armed with the capabilities to maintain optimum process settings and prevent similar problems in the future.
The end results were indeed impressive: a 65% reduction in the amounts of toxic side products – which translated into annual savings of one million euros for that single production line.