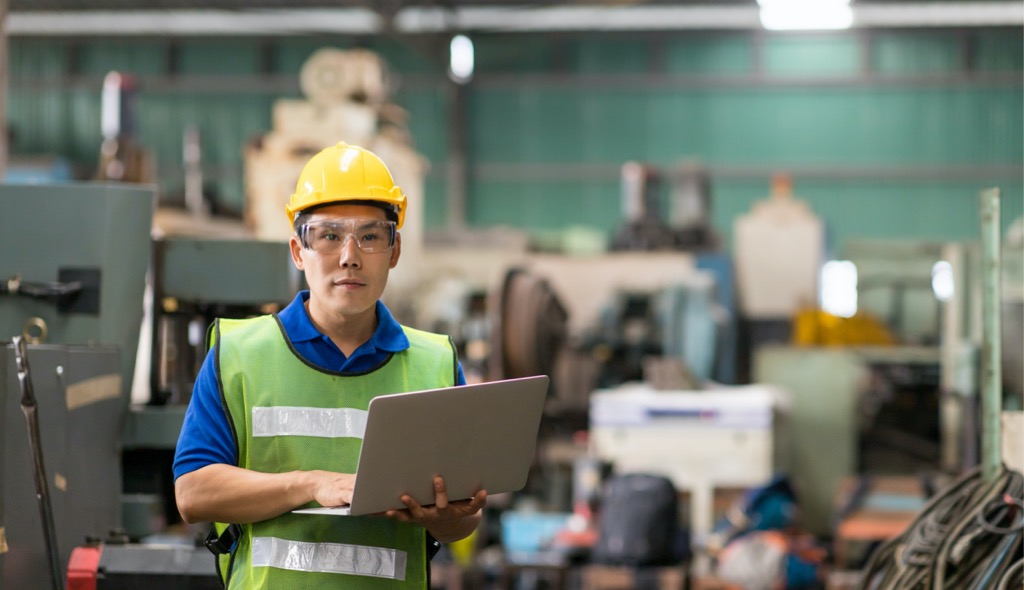
With more and more advancements in technology, implementing an achievable process optimization plan is no longer farfetched. However, you first need to find the right technologies and approaches that work best for your particular manufacturing operation.
The key to optimizing a manufacturing process is to embrace some of the advanced industry 4.0 technologies available today. By understanding which technology is best for your manufacturing business, you will be one step closer to optimizing your process. Let’s dive a bit deeper into what this means, and into the four main technology pillars to process optimization in manufacturing.
1) Leverage Real-Time Data By Adopting Industry 4.0 Technologies
The implementation of automation and use of data in manufacturing is what’s called “Industry 4.0″, with use cases such as predictive maintenance and predictive quality. Industry 4.0 includes the following technologies critical to process optimization:
- Real-time data connectivity and capture – Use industrial IoT connectivity to securely connect to the production line assets and capture data in a central time-series repository – either on-premise or on-cloud.
- Process-based machine learning – Use process-based artificial intelligence to get visibility into the full manufacturing process in detail, and holistically, and to discover and surface process issues that need attending. By using machine learning algorithms to process and analyze real-time data, not only can process inefficiencies be identified, but they can be predicted and even avoided.
- Digital Twin visualization – A digital twin is a virtual representation that matches the attributes and operational metrics of a “physical” production line through the captured production-line data. A digital twin of the production line enables you to quickly pinpoint performance anomalies and their root cause, providing you with actionable insights, and presenting them in the context of the production line. By having this ability, there is no need for data scientists – the system is easy-to-use and accessible for production teams.
2) Discover Primary Causes Of Process Inefficiencies
As mentioned above, by implementing process-based artificial intelligence, process engineers can identify inefficiencies, such as the formation of undesired side products, process instabilities, impurities and more. This can be done with Automated Root Cause Analysis.
Before understanding how this will help you achieve process optimization, let’s take a look at the difference between traditional root cause analysis, and automated root cause analysis.
Firstly, traditional root cause analysis takes time – often measured in days – and expert resources from multiple teams. With massive amounts of data captured from thousands of tags every minute, it’s almost impossible to find correlations between the operational variables that lead to a process inefficiency. The longer the analysis takes – the longer the process inefficiency happens in the production line.
For this reason, production teams need a faster and more accurate way of finding early events that lead to production failures.
Automated root cause analysis enriches historical and real-time asset data, and applies machine learning algorithms to automatically trace the causal chain of events leading to production failures.
By doing so, investigation teams get fast and accurate insight into early symptoms of process inefficiencies, making it easy for them to pinpoint and mitigate the root causes.
3) Predict When Process Inefficiencies Will Happen
Having the ability to identify why process inefficiencies in your production line happen, is priceless. But if you take this one step forward, you can also anticipate exactly when they will happen.
By applying industrial predictive analytics, you have the ability to translate data into predictive insights.
Machine learning algorithms can then be implemented to identify relevant events and predict their outcomes.
For example, predicting when undesired side products will form, or when a specific process instability will happen. By doing this, process teams are able to increase yield and prevent imminent quality failures.
4) Determine The Best Fit Process Values To Avoid Process Inefficiencies
Once we’ve understood why process inefficiencies happen and can predict them before they happen, it is fundamental to understand how to optimize the manufacturing process with these insights at hand.
Predictive simulation determines how specific inefficiencies can be avoided by simulating how processes will behave in different scenarios, and how to avoid the anticipated process inefficiency.
By using predictive simulation, process teams can:
- Close the loop and take action on analytics recommendations
- Adjust only the production settings that will eliminate process inefficiencies
- Reduce the risks in mis-adjusting production settings
To summarize, the coming of age of industrial artificial intelligence, and machine learning specifically, has introduced an opportunity to harness production-line data to surface actionable insights and drive continuous improvement in manufacturing processes. And digital twin visualization makes it now possible for process engineering teams to use these insights independently of data scientists and take action in a timely manner.
Ready to get started with process optimization, driven by data and machine learning? Contact us!