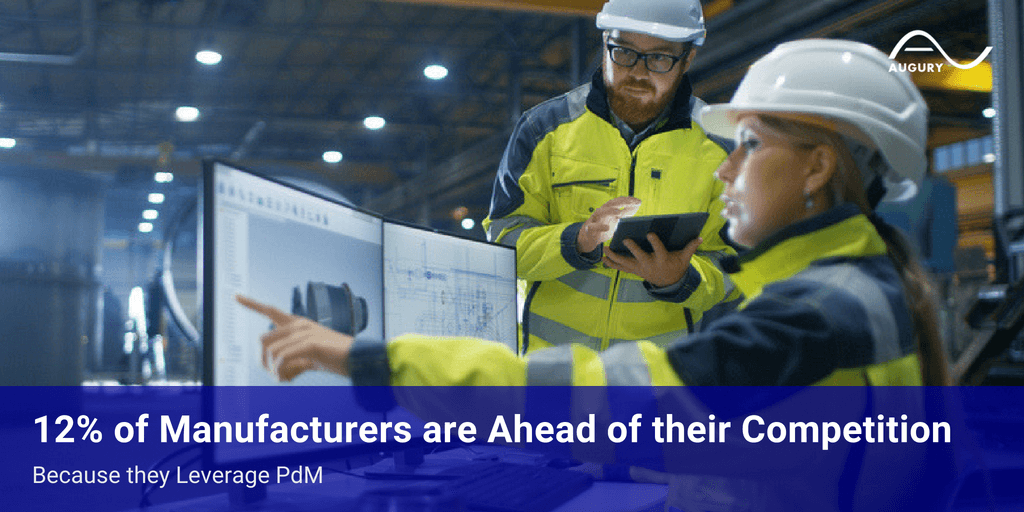
If you oversee a commercial or industrial operation that relies on motors, chances are you’d like to have a more predictive maintenance program. This sentiment rings true universally among engineering and maintenance leaders, yet only 12 percent of organizations that stand to benefit from predictive maintenance (PdM) employ the practice.
If you oversee a commercial or industrial operation that relies on motors, chances are you’d like to have a more predictive maintenance program. This sentiment rings true universally among engineering and maintenance leaders, yet only 12 percent of organizations that stand to benefit from predictive maintenance (PdM) employ the practice.
While recent advancements in technology make PdM more accessible and effective than ever, the “when” and “how” of employing new maintenance technologies constitutes a common problem for both commercial and industrial operations. This lack of adoption stems not from skepticism regarding PdM’s utility, but from skepticism regarding its return on investment.
To illustrate why, let’s consider the market’s common experience with traditional predictive maintenance tools and services. Of the organizations I’ve spoken with that use predictive maintenance techniques, most do so on a limited basis – which makes sense considering that traditional PdM techniques require time to collect and analyze machine data, limiting the frequency and accessibility of their assessments. Likewise, internal PdM programs are often limited due to the expense of the tools, expertise and manpower required for execution.
Implementing vibration analysis, generally accepted as the most effective method of PdM for rotating equipment, requires first using a sensor to record the vibrations emanating from a piece of rotating equipment, and then interpreting those vibrations in the form of a complicated sine graph. Many vibration recording tools are expensive and require extensive training to use – and that’s just to enable the collection of vibration data. Analyzing that data requires a distinct and costly expertise.
When considering the potential ROI in utilizing predictive maintenance techniques, most organizations choose either to not implement or to implement with compromises. Here’s the common thread throughout the many conversations I’ve had with maintenance leaders implementing an internal predictive maintenance solution:
Most have trained a few technicians to record machine data during maintenance routes, and they catch some issues by tracking trends in the data. Once in a while, they send data out for further analysis. The majority of them still have a lot of reactive moments. Most understand the potential value of predictive maintenance techniques at scale, but they don’t feel that they have the resources to grow a program.
In these cases, the inability to effectively scale the use of predictive techniques results in a schedule-based preventive maintenance program that doesn’t monitor the condition of machines continuously enough to reach proactivity. While these maintenance leaders recognize the value of predictability, their limited results breed skepticism concerning the investment required to reach that predictability.
So how do you reach true predictability at a relatively reasonable cost?
If predictability requires knowing the condition of assets before failures occur, then the ultimate route to predictability at scale is continuous diagnostics. Continuous diagnostics combines continuous data collection and continuous analysis of that data. If you can identify specific mechanical issues early enough, then you can go further than preventing asset failure – you can prevent machine degradation in the first place. And if you can do that, investment in the technology that makes it possible becomes much less speculative.
You might have familiarity with continuous data collection through the use of sensors on the bearing points of machines, but what about continuous analysis? By applying machine learning technology, some predictive maintenance companies are now training artificially intelligent algorithms to assist vibration analysis, and an advanced AI can enable diagnostics that occur almost as fast as data collection. The results are continuous, actionable insights into machine health delivered to stakeholders in real time – no training or expertise required.
Here’s an example of how this type of AI-driven technology plays out in an industrial manufacturing context:
Imagine sensors installed on each motor and gearbox in a full production line. These sensors measure vibration, temperature, and magnetic flux, and continuously feed that data into the cloud where well-trained algorithms analyze it in real time. A vibration anomaly is detected, triggering the algorithm to compare current data against a trove of historical data from that machine and thousands of others like it. Minutes after detecting the vibration anomaly, algorithms identify a worn bearing on the driven side of a motor driving a critical component of the line. The maintenance team receives a notification of this precise issue and plans a shutdown to replace the bearing. Upon further inspection, technicians discover damage so severe that had the repair not been immediate, the issue would have soon taken down the entire line.
What are the financial implications of this example? By identifying the precise mechanical issue at such an early stage, a maintenance team can plan the repair, which in this case, requires only the replacement of a bearing. Without continuous diagnostics, that bearing would have quickly caused a misalignment or worse, resulting in damage to other parts of the machine and ultimately a failure. The ability to know not only that a vibration anomaly occurred, but why it occurred, saves this team on parts, labor, time, and a sizable amount of production. At scale, this solution optimizes PM routes and machine efficiency, substantially reducing parts inventory and extending asset life. For some of Augury’s partners, one save like this covers the cost of having continuous diagnostics on a production line for one year.
By using advanced predictive maintenance technology to eliminate the time and expertise required by traditional techniques, maintenance leaders achieve a much greater return on a much lower investment. So if you share the dream of predictability for your maintenance program, as well as the skepticism concerning its ROI, consider reevaluating the market. As technology begins to deliver on the promise of predictability, early adopters stand to gain a particularly competitive edge.
Looking into implementing Predictive Maintenance or updating your current PdM program? Get in touch.