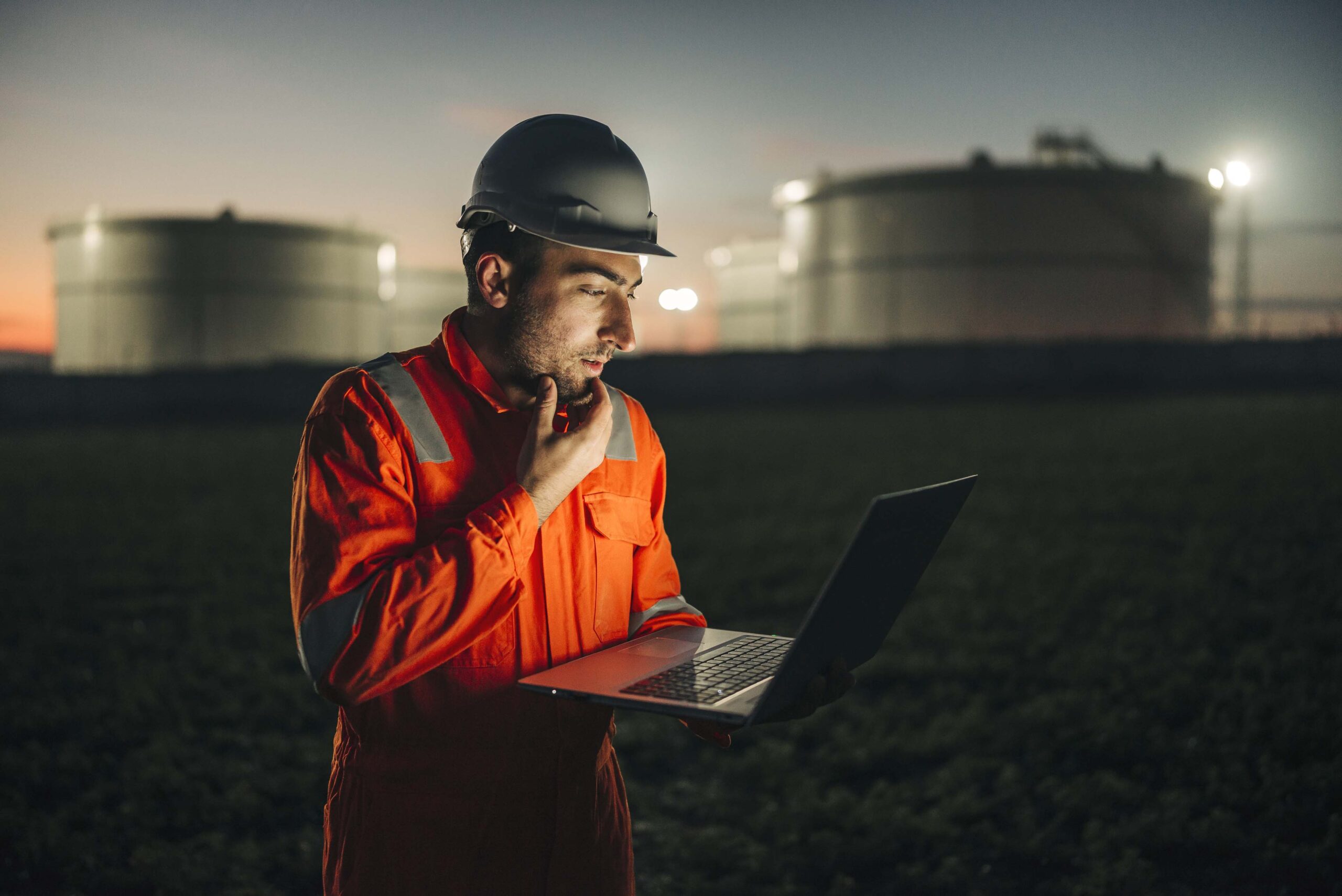
With production optimization as the key route to increasing revenue and reducing costs, chemical manufacturers are increasingly turning to AI-driven solutions to keep assets running and streamline process.
One of the main ongoing concerns for manufacturers is how to enhance productivity. This includes analyzing, measuring, modeling and implementing specific actions to optimize their production lines.
The reason production optimization plays a huge role in manufacturing, is because it is a core means to increase revenue and reduce costs. Process failures in production cause companies to suffer from losses in quality and yield – which translate directly into revenue loss.
Let’s take a look at the chemical manufacturing industry, as the birth of the heavy chemical industry coincides with the beginning of the Industrial Revolution. The chemical industry comprises about 15% of the US manufacturing sector, manufactures more than 70,000 different products, and is responsible for 90% of our everyday products.
Challenges Chemical Manufacturers Face
Just as broad as the chemical manufacturing industry is, so are the process optimization challenges it faces.
In order for chemical manufacturers to optimize production lines, they need to address different process inefficiencies, such as the formation of undesired side products, process instabilities, losses due to impurities and more, on an ongoing basis.
Given the complexities of chemical manufacturing, it’s extremely time-consuming and difficult to understand the root causes for these process inefficiencies, let alone anticipate when they are going to happen. Often times, it is the specific behavior of the combination of multiple production parameters, or tags, that cause the inefficiency to happen.
A growing number of chemical manufacturers are turning to Industrial Artificial Intelligence solutions to identify and anticipate process inefficiencies leveraging methods of supervised and unsupervised machine learning.
Proven Success Story
According to recent research by Accenture, companies that have implemented Industrial AI in the chemical sector are seeing big benefits—a whopping 72 percent report a minimum 2x improvement in some process KPIs, and 37 percent a 5x improvement. For example, a manufacturer of Ethylene Dichloride implemented process-based Industrial AI to solve a number of process inefficiencies, and by doing so increased yield by €1.7M in less than 12 months.
With the capabilities Industrial AI has to offer, chemical manufacturers can utilize their data, improve their processes, and continually adapt them.
Revolutionizing the Chemical Manufacturing Industry
Chemical manufacturers need to identify and avoid process inefficiencies to improve chemical process control.
A production disturbance is any unintentional event in the chemical production process that leads to process inefficiencies, unplanned stoppages, rework, or scrap
By implementing Industrial AI solutions to chemical production lines, manufacturers have the ability to leverage different AI technologies that are critical to identifying production disturbances and optimizing production:
- Real-time data connectivity and capture – manufacturers use industrial IoT connectivity to securely connect to the production line assets and capture data in a central time-series repository
- Process-based machine learning – manufacturers use process-based AI to get visibility into the full manufacturing process in detail, and holistically, and to discover and surface process issues that need attending.
- Digital Twin visualization – manufacturers use a digital twin, which is a virtual representation that matches the attributes and operational metrics of a “physical” production line through the captured production-line data. This enables production teams to quickly pinpoint performance anomalies and their root cause, providing them with actionable insights, and presenting them in the context of the production line. This eliminates the need for data scientists.
How to Use AI for Predictive & Prescriptive Process Optimization
Let’s dive a bit deeper into how specific Industrial AI technologies can be used to identify, anticipate, and prevent chemical process inefficiencies:
- Implement Digital Twin Visualization
The first step manufacturers should take to identify specific process inefficiencies is implementing digital twin visualization. This allows them to easily track their main KPIs and receive actionable insights into process anomalies.
- Perform Automated Root Cause Analysis
Automated Root Cause Analysis can then be performed to gain fast and accurate insights into process inefficiencies. This approach enriches historical and real-time asset data and applies machine learning algorithms to automatically trace the causal chain of events leading to production failures.
- Translate Data into Insights with Industrial Predictive Analytics
Once process inefficiencies have been identified using the analyzed data, it’s important to translate this data into actionable insights. Industrial Predictive Analytics can achieve this.
Machine learning algorithms can be implemented to identify relevant events and predict their outcomes.
By having the ability to prevent specific inefficiencies and production disturbances, process teams can increase production yield while preventing failures at the same time.
Bottom Line: Save Time And Money
By using process-based machine learning, manufacturers get focused and contextual predictive alerts. This is a huge opportunity for chemical manufacturers, since operational technology (OT) data is already well organized and captured within data historians.
Leveraging this data with process-based AI means being able to pinpoint the root cause of process disturbances with extreme accuracy, and predict process instabilities and failures before they have the chance to affect production.
So, with Industrial AI, chemical manufacturers can reduce quality and production losses, saving them great amounts of time and money.
Ready to get started with process optimization, driven by data and machine learning? Reach out.